UGent Data Science Seminar with Prof. Nathalie Pochet (Broad & Harvard med school)
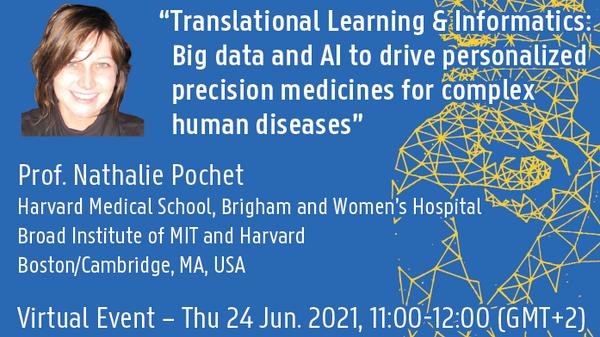
Speaker: Prof. Nathalie Pochet
Harvard Medical School, Brigham and Women’s Hospital
Broad Institute of MIT and Harvard
Title: Translational Learning & Informatics: Big data and AI to drive personalized precision medicines for complex human diseases
Abstract
I will talk about the engineering and AI-driven algorithms and software tools that I (co-)developed, including M@CBETH, SERV, MetageneProjection, GenomeSpace, Trinity, Trinity-CTAT, TFUtils, *AMARETTO and AMARETTO-Hub, all critical tools that have significantly helped advance and overcome challenges in biomedical research for better understanding, diagnosis and treatment of human disease. For example, my tool SERV led to my fine mapping of the highly-elusive causal genetic variant for the rare dominant Mendelian kidney disease ‘medullary cystic kidney disease type 1’ (MCKD1), which was then extensively confirmed experimentally using an assay that allows for screening and alleviates challenges of living-relative kidney donation. Trinity has led to the initial characterization of the SARS-CoV-2 viral sequence and its abundance estimation. Recently, my *AMARETTO and AMARETTO-Hub tools led to my predicting drug treatments for chemoprevention of hepatocellular carcinoma of viral and other etiologies, including hepatitis C and B virus-induced hepatocellular carcinoma, and experimental validation confirmed that two novel predicted compounds indeed attenuate liver fibrosis and cancer development in rats, and thus, potentially offer a safe and low-cost approach for chemoprevention of hepatitis C and B virus-infected patients who are at greater risk of progressing to hepatocellular carcinoma. These and other examples emphasize the power of my computational approaches. I will explain how I solved these problems by using a variety of “modern” AI techniques, including concepts from transfer learning, (un)supervised machine learning, interpretable learning, multimodal and multiscale data fusion, network biology and medicine, knowledge graphs, and active learning.
Bio
I am a multidisciplinary scientist with expertise in developing innovative computational approaches for studies of human disease. I received an extensive and unusually broad training resulting in three M.Sc. degrees in the fields of Engineering Sciences in Electrical Engineering and Computer Science, Artificial Intelligence, and Bioinformatics, and a Ph.D. degree in Engineering Sciences in Machine Learning and Bioinformatics. Training with leaders in the fields of machine learning, computer science, statistics, biology, genomics and medicine has been instrumental in shaping my thinking as a multidisciplinary scientist. My lab in “Translational Learning & Informatics” at the Broad Institute of MIT and Harvard and Harvard Medical School focuses on the development of engineering and AI-driven algorithms and software tools for better diagnosis and therapy in human disease, including cancer, infectious, neurological and immune-mediated diseases. My work across model systems and patients in studies of human disease led to groundbreaking discoveries in fundamentals of evolution, the rare Mendelian kidney disease MCKD1, the human malaria parasite Plasmodium falciparum, and chemoprevention of viral and lifestyle etiologies of cancer. I also act as a Principal Investigator in the NIH NCI’s Informatics Technology for Cancer Research Program that focuses on the development and dissemination of algorithms and software tools, and their translation to clinical practice and industrial partnerships.
The seminar will be organized online or hybrid, depending on the COVID situation at the time of the seminar. The decision will be made one week in advance, and the online participation link will be sent to all registered participants at that time.
Location and time
Thursday, June 24, 2021 11:00 AM to 1:00 PM GMT+2 online event
Register for the event on Meetup to get the link to this online event.